30 November 2022
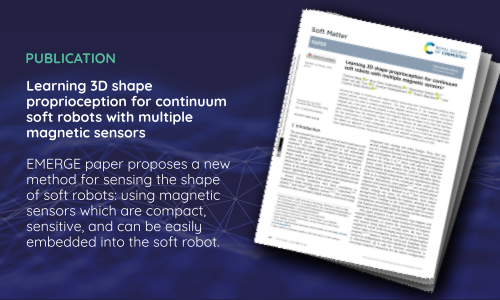
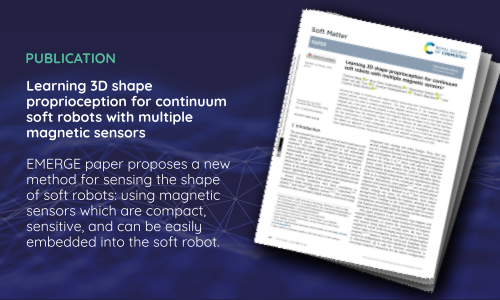
Soft robots are made of materials similar to living tissues, such as elastomers and gels, which allow them to deform their bodies, adapt to their environment, and manipulate objects. For that reason soft robotics is a rapidly growing field with a wide range of applications, including food and beverage processing, laparoscopic surgery, prosthetics, disaster relief, and even space exploration. At the same time, they are more complex to model, control, and sense than rigid robots.
Sensing the shape of soft robots is an especially intricate task. Sensors must not obstruct the natural behaviour and movement of the robots or reduce their natural softness. At the same time, non-collocated and nonlinear sensors require algorithms for the measurements to be interpreted and connected to a description of the robot's shape. As such it requires innovative technological and algorithmic solutions
In their paper published in the journal Soft Matter, EMERGE partners from the Delft University of Technology (NL) and collaborators propose a new method for sensing the shape of soft robots: using magnetic sensors which are compact, sensitive, and can be easily embedded into soft robots.
The researchers also developed a neural network-based architecture that learns to estimate the robot’s 3D shape from the sensor readings and incorporates prior knowledge of the robot’s deformation modes. The approach allows for the rearrangement and removal of redundant sensors without requiring retraining of the neural network. The method was validated through simulations and experiments, achieving a mean relative error of 4.5%.
Source: T. Baaij, M. Holkenborg, M. Stölzle, D. van der Tuin, J. Naaktgeboren, R. Babuška, and C. Della Santina, “Learning 3D shape proprioception for continuum soft robots with multiple magnetic sensors,” Soft Matter, vol. 19, no. 1, pp. 44–56, 2023, DOI: 10.1039/D2SM00914E.
Access EMERGE publications in the link below.